import pandas as pd
= "https://raw.githubusercontent.com/fahadsultan/csc272/main/data/elections.csv"
url
= pd.read_csv(url) elections
Filtering: subset of rows
Extracting a subset of rows from a DataFrame
is called filtering.
We can filter rows based on a boolean condition, similar to conditional statements (e.g., if
, else
) in Python.
For example, to filter rows of candidates who ran for elections since 2010, we can use the following code:
First k rows
The .head()
method is used to view the first few rows of a DataFrame. By default, it returns the first 5 rows.
elections.head()
Year | Candidate | Party | Popular vote | Result | % | |
---|---|---|---|---|---|---|
0 | 1824 | Andrew Jackson | Democratic-Republican | 151271 | loss | 57.210122 |
1 | 1824 | John Quincy Adams | Democratic-Republican | 113142 | win | 42.789878 |
2 | 1828 | Andrew Jackson | Democratic | 642806 | win | 56.203927 |
3 | 1828 | John Quincy Adams | National Republican | 500897 | loss | 43.796073 |
4 | 1832 | Andrew Jackson | Democratic | 702735 | win | 54.574789 |
Last k rows
The .tail()
method is used to view the last few rows of a DataFrame. By default, it returns the last 5 rows.
elections.tail()
Year | Candidate | Party | Popular vote | Result | % | |
---|---|---|---|---|---|---|
177 | 2016 | Jill Stein | Green | 1457226 | loss | 1.073699 |
178 | 2020 | Joseph Biden | Democratic | 81268924 | win | 51.311515 |
179 | 2020 | Donald Trump | Republican | 74216154 | loss | 46.858542 |
180 | 2020 | Jo Jorgensen | Libertarian | 1865724 | loss | 1.177979 |
181 | 2020 | Howard Hawkins | Green | 405035 | loss | 0.255731 |
Random k rows
The .sample()
method is used to view a random sample of rows from a DataFrame. By default, it returns a single random row.
elections.sample()
Year | Candidate | Party | Popular vote | Result | % | |
---|---|---|---|---|---|---|
162 | 2008 | Barack Obama | Democratic | 69498516 | win | 53.02351 |
.sample()
method can also be used to return multiple random rows by specifying the number of rows to return using the n
parameter.
7) elections.sample(
Year | Candidate | Party | Popular vote | Result | % | |
---|---|---|---|---|---|---|
111 | 1960 | John Kennedy | Democratic | 34220984 | win | 50.082561 |
20 | 1856 | James Buchanan | Democratic | 1835140 | win | 45.306080 |
67 | 1912 | Eugene W. Chafin | Prohibition | 208156 | loss | 1.386325 |
50 | 1896 | John M. Palmer | National Democratic | 134645 | loss | 0.969566 |
32 | 1872 | Ulysses Grant | Republican | 3597439 | win | 55.928594 |
101 | 1948 | Henry A. Wallace | Progressive | 1157328 | loss | 2.374144 |
14 | 1848 | Lewis Cass | Democratic | 1223460 | loss | 42.552229 |
Filtering by position
The []
selection operator is the most baffling of all, yet the most commonly used. It only takes a single argument: A slice of row numbers
Say we wanted the first four rows of our elections
DataFrame.
0:4] elections[
Year | Candidate | Party | Popular vote | Result | % | |
---|---|---|---|---|---|---|
0 | 1824 | Andrew Jackson | Democratic-Republican | 151271 | loss | 57.210122 |
1 | 1824 | John Quincy Adams | Democratic-Republican | 113142 | win | 42.789878 |
2 | 1828 | Andrew Jackson | Democratic | 642806 | win | 56.203927 |
3 | 1828 | John Quincy Adams | National Republican | 500897 | loss | 43.796073 |
Filtering by condition
Step 1. A Filtering Condition
Perhaps the most interesting (and useful) method of selecting data from a Series is with a filtering condition.
First, we apply a boolean condition to the Series. This create a new Series of boolean values.
= pd.Series({'a': 1, 'b': 2, 'c': 3, 'd': 4})
series > 2 series
a False
b False
c True
d True
dtype: bool
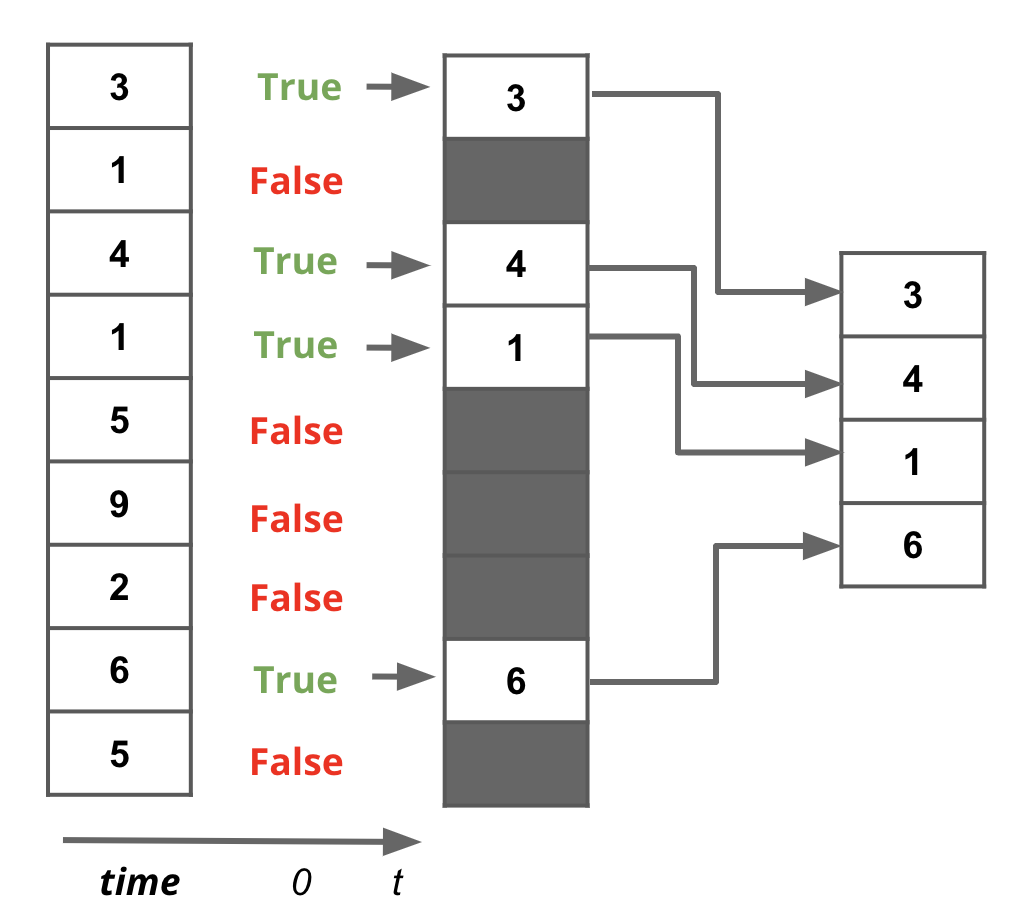
We then use this boolean condition to index into our original Series. pandas will select only the entries in the original Series that satisfy the condition.
= series > 2
condition1 = elections['Year'] > 2010 condition2
Step 2. Applying the Condition
The second step is to apply the condition (pd.Series of boolean values, equal to the length of the DataFrame) to the DataFrame using the []
operator.
series[condition1]
c 3
d 4
dtype: int64
elections[condition2]
Year | Candidate | Party | Popular vote | Result | % | |
---|---|---|---|---|---|---|
168 | 2012 | Barack Obama | Democratic | 65915795 | win | 51.258484 |
169 | 2012 | Gary Johnson | Libertarian | 1275971 | loss | 0.992241 |
170 | 2012 | Jill Stein | Green | 469627 | loss | 0.365199 |
171 | 2012 | Mitt Romney | Republican | 60933504 | loss | 47.384076 |
172 | 2016 | Darrell Castle | Constitution | 203091 | loss | 0.149640 |
173 | 2016 | Donald Trump | Republican | 62984828 | win | 46.407862 |
174 | 2016 | Evan McMullin | Independent | 732273 | loss | 0.539546 |
175 | 2016 | Gary Johnson | Libertarian | 4489235 | loss | 3.307714 |
176 | 2016 | Hillary Clinton | Democratic | 65853514 | loss | 48.521539 |
177 | 2016 | Jill Stein | Green | 1457226 | loss | 1.073699 |
178 | 2020 | Joseph Biden | Democratic | 81268924 | win | 51.311515 |
179 | 2020 | Donald Trump | Republican | 74216154 | loss | 46.858542 |
180 | 2020 | Jo Jorgensen | Libertarian | 1865724 | loss | 1.177979 |
181 | 2020 | Howard Hawkins | Green | 405035 | loss | 0.255731 |
To filter rows based on multiple conditions, we can use the &
operator for AND
and the |
operator for OR
.
For example, to filter rows of candidates who won the elections with less than 50% of the votes, we can use the following code:
= (elections['Result'] == 'win') & (elections['%'] < 50)
condition
elections[condition]
Year | Candidate | Party | Popular vote | Result | % | |
---|---|---|---|---|---|---|
1 | 1824 | John Quincy Adams | Democratic-Republican | 113142 | win | 42.789878 |
16 | 1848 | Zachary Taylor | Whig | 1360235 | win | 47.309296 |
20 | 1856 | James Buchanan | Democratic | 1835140 | win | 45.306080 |
23 | 1860 | Abraham Lincoln | Republican | 1855993 | win | 39.699408 |
33 | 1876 | Rutherford Hayes | Republican | 4034142 | win | 48.471624 |
36 | 1880 | James Garfield | Republican | 4453337 | win | 48.369234 |
39 | 1884 | Grover Cleveland | Democratic | 4914482 | win | 48.884933 |
43 | 1888 | Benjamin Harrison | Republican | 5443633 | win | 47.858041 |
47 | 1892 | Grover Cleveland | Democratic | 5553898 | win | 46.121393 |
70 | 1912 | Woodrow Wilson | Democratic | 6296284 | win | 41.933422 |
74 | 1916 | Woodrow Wilson | Democratic | 9126868 | win | 49.367987 |
100 | 1948 | Harry Truman | Democratic | 24179347 | win | 49.601536 |
117 | 1968 | Richard Nixon | Republican | 31783783 | win | 43.565246 |
140 | 1992 | Bill Clinton | Democratic | 44909806 | win | 43.118485 |
144 | 1996 | Bill Clinton | Democratic | 47400125 | win | 49.296938 |
152 | 2000 | George W. Bush | Republican | 50456002 | win | 47.974666 |
173 | 2016 | Donald Trump | Republican | 62984828 | win | 46.407862 |
.drop_duplicates()
If we have a DataFrame with many repeated rows, then .drop_duplicates()
can be used to remove the repeated rows.
Where .unique()
only works with individual columns (Series) and returns an array of unique values, .drop_duplicates()
can be used with multiple columns (DataFrame) and returns a DataFrame with the repeated rows removed.
'Party']].drop_duplicates() elections[[
Party | |
---|---|
0 | Democratic-Republican |
2 | Democratic |
3 | National Republican |
6 | Anti-Masonic |
7 | Whig |
15 | Free Soil |
21 | Republican |
22 | American |
24 | Constitutional Union |
25 | Southern Democratic |
26 | Northern Democratic |
27 | National Union |
31 | Liberal Republican |
35 | Greenback |
38 | Anti-Monopoly |
41 | Prohibition |
42 | Union Labor |
48 | Populist |
50 | National Democratic |
58 | Socialist |
68 | Progressive |
78 | Farmer–Labor |
89 | Communist |
93 | Union |
103 | Dixiecrat |
110 | States' Rights |
115 | American Independent |
121 | Independent |
125 | Libertarian |
127 | Citizens |
136 | New Alliance |
147 | Taxpayers |
148 | Natural Law |
149 | Green |
150 | Reform |
160 | Constitution |